In addition to its impressive sensitivity, liquid chromatography-tandem mass spectrometry (LC-MS/MS) analysis brings the advantage of automatic metadata collection. This analytic information about the observed peak gives labs insight into performance of the chromatography, MS, sample preparation, and usually some combination of all three. Ultimately, laboratories use metadata to decide whether a result is reportable or needs further investigation.
Typically labs analyze metadata during method development and validation to compare against published acceptance criteria such as the Clinical and Laboratory Standards Institute guideline, CLSI-C62A. Guideline criteria come from expert opinion, consensus debate, and an appreciation for state of the art in MS instrumentation.
Often, labs only apply metadata in the per-sample or per-batch context after validation. However, once a method is in production, the true power of metadata comes into play. High-throughput laboratories test hundreds of thousands of samples per year. Yet typical clinical LC-MS/MS testing isolates those data sets at the batch level, limiting the power of statistical analysis. Software developed at Indigo BioAutomation enables users to analyze in a unified database de-identified, high-throughput production data. This facilitates statistical evaluation of very large data sets for method stability and comparison of production-level performance to acceptance criteria. In this article, we show metadata examples from approximately 2.5 million samples tested over 7 months across various laboratory types and sizes.
We removed from our analysis those samples without signal: blanks, double blanks, and undetected results. We also removed results from those labs with an average production rate of less than one 96-well plate per day. We intentionally retained re-injected or rejected samples in order to maximize our awareness of the full data range.
Retention Time Variance
Our first example is the observed retention time (Rt) for 3,4-methylenedioxymethamphetamine (MDMA) from four separate methods. In this context, a method refers to one LC-MS/MS system (Figure 1). The dashed lines are ±0.15 minutes around the median calculated for the 200 day interval. The grey bar is ±2.5% of the median (CLSI-C62A recommended tolerance).
Since Rt primarily reflects chromatography, this graph monitors the state of the LC column, pumps, and plumbing. The red and cyan methods are highly reproducible, while the blue drifts to a lower Rt, and the magenta has both a repeating drift pattern and large, episodic spikes. The maintenance logs for the magenta method might reveal whether these patterns correlate to LC column or mobile phase changes. Alternatively, the magenta LC or sample preparation protocols may lack robustness, such that small variances in process yield large Rt shifts.
Typically, the tolerance range is referenced to the mean Rt of calibrators within a batch. That may be sufficient to bring all the methods within recommended thresholds, but note that the cyan instrument meets CLSI-C62A-recommended tolerance 96% of the time using the global median.
Internal Standard Peak Area Variance
Our second example is the observed peak area for deuterated codeine internal standard (IS). We scaled the areas from each method in order to compare the variance between methods. The means are shown with a solid line (Figure 2). The lighter shade marks variance at ±2 standard deviations, and the dashed lines define ±50% of the mean (a common acceptance criteria). Because IS is added uniformly to every sample, IS peak area consistency tells us something about the precision of IS addition as well as chromatography and MS/MS status. IS variance also indicates whether the sample preparation has standardized ion suppression between samples.
Notably, of the three methods, only the blue is able to provide a consistent measure. The red shifts at index 22000 to a higher area and wider variance, while the green shows one slight shift in the average along with two increases in variance. All three methods have a brief variance spike—indicated by the solid arrow—before returning to the prior mean. This change is not detected using the ±50% acceptance criteria, suggesting that variance is more informative.
Some change in the mean value is anticipated, for example, by new IS lots or declining MS/MS response with use and rebound after cleaning. However, this would not explain the large changes in IS variance within the red and green systems. As with Rt, using a within batch reference point (mean of the calibrator IS response) is standard practice to correct for between batch variance. However, monitoring IS response longitudinally, as is done here, often provides an early alert for method problems. With proactive detection and intervention, batch failures, reporting delays, and unplanned instrument down-time can be minimized.
MRM or Ion Ratio (ION-R) Variance
Our final example characterizes the variance in ION-R for oxymorphone and lorazepam (Figure 3). All positive samples (n > 300,000) were characterized as passing or failing at one of eight ION-R tolerance ranges. We display the median (bold line), 25th/75th percentile (box) and outlier-adjusted min/max (whisker) for ION-R acceptance rate/lab at each tolerance range (Figure 4). Red circles are outliers. We arbitrarily defined success as a 95% acceptance rate/lab (horizontal blue line) and look for the tolerance threshold where the box meets this acceptance rate.
The tolerance level (x-axis) is the acceptable percent difference from the mean ION-R value, while the acceptance rate (y-axis) is the percent of samples from any given lab which are within that tolerance. The mean ION-R of each batch is calculated using every sample which generates both quantifier and qualifier signal. The cumulative acceptance rate for each lab, across all batches for that lab, was then calculated. Thus, each laboratory provides a single data point for each box of the box plot.
To further clarify the nature of a box plot, for oxymorphone at the 5% tolerance level we have overlaid a bar graph for eight imaginary labs in the distribution. Lab A has the median acceptance rate, labs B and G have the maximum and minimum acceptance rates respectively, and labs D and E have acceptance rates at the 75th and 25th percentile respectively. Outliers are identified by their distance from the 25th percentile, and based on the length of the box. Since the box defines the inner quartile range (IQR, middle 50% of all values), a lab is considered an outlier if it’s acceptance rate is more than one and a half IQRs below the 25th percentile.
ION-R is complex metadata, dependent on both quantifier and qualifier peak areas, which may have different susceptibilities to method variance. Nearly 75% of labs are successful when measuring oxymorphone with a ±25% tolerance. For lorazepam, similar success requires a ±40% tolerance and even at the widest tolerance (±50%) there are labs with unusually low acceptance rates. Low acceptance rates may indicate insufficient signal from an MS/MS needing maintenance, a low abundance product ion, frequent co-eluting interferences, or dramatic differences between the ION-R in standards versus unknowns. A time series, similar to the prior graphs, might reveal temporal fluctuations related to instrument status.
Conclusion
Clinical laboratories using LC-MS/MS need to strike a balance between the maximum achievable quality and quality that is sufficient for purpose. The high level analysis we present here intentionally ignores the extenuating circumstances surrounding any one method. Nevertheless, we draw three important conclusions. First, it appears unlikely that all laboratories are meeting the consensus guidelines consistently. Second, the consensus guidelines are clearly achievable. Third, metadata monitored over the long term per method is a powerful quality assurance resource. We see this type of analysis as a valuable tool for individual laboratories and also for regulatory and consensus groups. “Big metadata” analysis of very large data sets serves as a practical reality check and as an adjunct to expert opinion.
Adam P.R. Zabell, PhD, is a product manager and scientist at Indigo BioAutomation in Indianapolis. +Email: [email protected]
Judith Stone, PhD, is the senior clinical laboratory scientist specialist at the University of San Diego toxicology laboratory in the Center for Advanced Laboratory Medicine and chair of CLN’s Focus on Mass Spectrometry editorial board. +Email: [email protected]
Randall K. Julian, PhD, is the CEO and founder of Indigo BioAutomation.
CLN's Focus on Mass Spectrometry is supported by Waters Corporation.
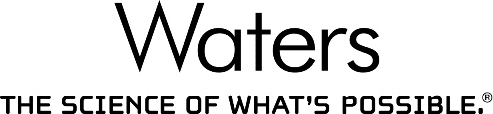